Date:
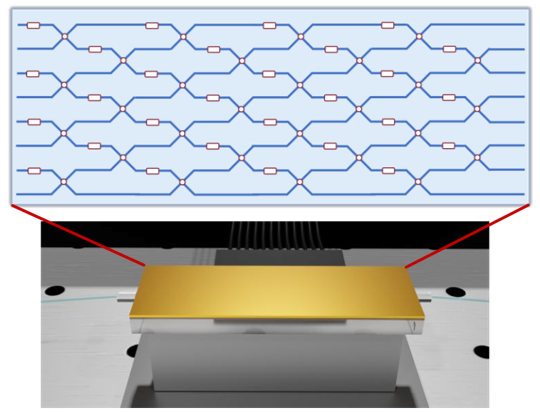
A new work published in Nature Communications, Quantum machine learning with Adaptive Boson Sampling via post-selectionNature Communications 16, 902 (2025), shows new applications of photonic Boson Sampling in quantum machine learning.
The work is the result of the collaboration between Sapienza, CNR-IFN and Leonardo within the NQSTI project.
The work aimed at investigating variants of the original Boson Sampling paradigm to find new applications of the protocol in the context of quantum computing and machine learning. In particular, the authors focused on the effects of adaptive measurements in the scheme. The goal of the experiment was to emulate in post-selection the effect of adaptive measurements, that is to detect part of the photons and apply different unitary transformations on the remaining photons according to the measurement’s outcomes. Such a novel paradigm was implemented in two different platforms: the first envisages a 6-mode universal circuit that processes photon pairs generated by a nonlinear crystal through spontaneous down-conversion, and the second envisages a quantum dot source, a synchronization stage to perform up-to 3-photon experiments, an 8-mode universal integrated circuit, and superconducting nanowire detectors. Such a scheme enabled the authors to encode classical data in the quantum system and to estimate quantum kernels that were employed by a standard support vector machine to solve a binary classification problem. The results confirmed the expressivity of the kernels retrieved through the adaptive Boson Sampling scheme. Furthermore, adaptive Boson Sampler devices promise to be applicable to other optimization tasks and, given the rapid development of integrated photonics and near-deterministic single-photon sources, to be applied more generally to other types of large-scale problems in the near future.
Reference: F. Hoch, E. Caruccio, G. Rodari, T. Francalanci, A. Suprano, T. Giordani, G. Carvacho, N. Spagnolo, S. Koudia, M. Proietti, C. Liorni, F. Cerocchi, R. Albiero, N. Di Giano, M. Gardina, F. Ceccarelli, G. Corrielli, U. Chabaud, R. Osellame, M. Dispenza, and F. Sciarrino, “Quantum machine learning with Adaptive Boson Sampling via post-selection”, Nature Communications 16, 902 (2025). https://doi.org/10.1038/s41467-025-55877-z
In the following image: - Adaptive Boson Sampling scheme for kernel estimation and binary classification. - Experimental photonic platform. - Structure of the optical circuit and experimental kernels.